What’s Next in AI is Fluid Intelligence
What’s Next in AI is Fluid Intelligence
Today's AI is narrow. Applying trained models to new challenges requires an immense amount of new data training, and time.
We need AI that combines different forms of knowledge, unpacks causal relationships, and learns new things on its own.
In short, AI must have fluid intelligence— and that's exactly what AI research teams are building.
Today's AI is narrow. Applying trained models to new challenges requires an immense amount of new data training, and time.
We need AI that combines different forms of knowledge, unpacks causal relationships, and learns new things on its own.
In short, AI must have fluid intelligence— and that's exactly what AI research teams are building.
Workstreams
Neurosymbolic AI
We're integrating neural and symbolic techniques to build AI that can perform complex tasks by understanding and reasoning more like we do.
AI Hardware
Our digital and analog accelerators are driving massive improvements in computational power while remaining energy-efficient.
Secure, Trusted AI
Trust and security should be baked into the core of any AI we put out into the world. We're building tools to help you ensure that it is.
AI Engineering
We're building tools to help AI creators reduce the time they spend training, maintaining, and updating their models.
Publication collections
Dec 2020 |
Conference on Neural Information Processing Systems (NeurIPS) |
|
Aug 2020 |
ACM SIGKDD International Conference on Knowledge Discovery and Data Mining (KDD) |
|
Jul 2020 |
Association for Computational Linguistics (ACL) |
|
Feb 2020 |
Association for the Advancement of Artificial Intelligence (AAAI) |
|
Dec 2019 |
Conference on Neural Information Processing Systems (NeurIPS) |
Featured
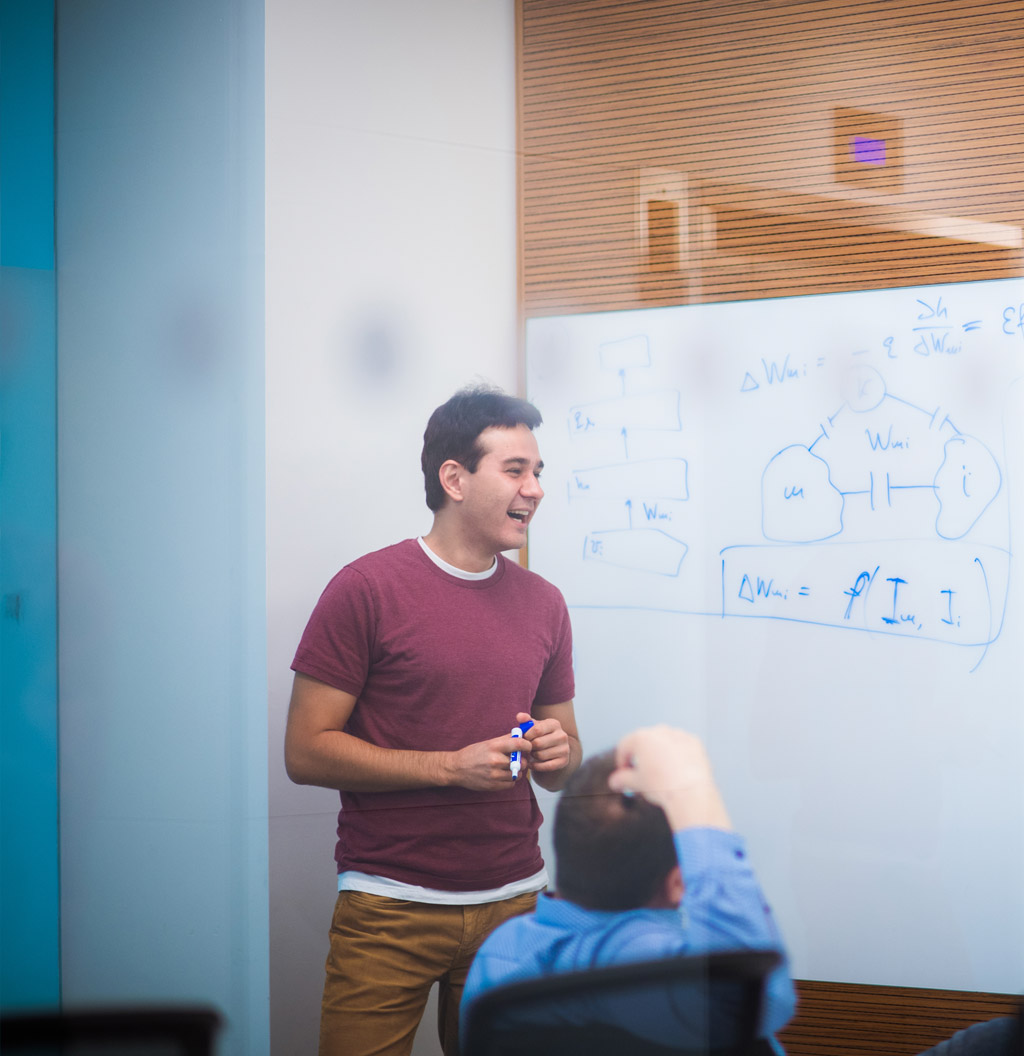
MIT-IBM
Watson AI Lab
We're partnering with the sharpest minds at MIT to advance AI research in areas like healthcare, security, and finance.
Recent news
Blog
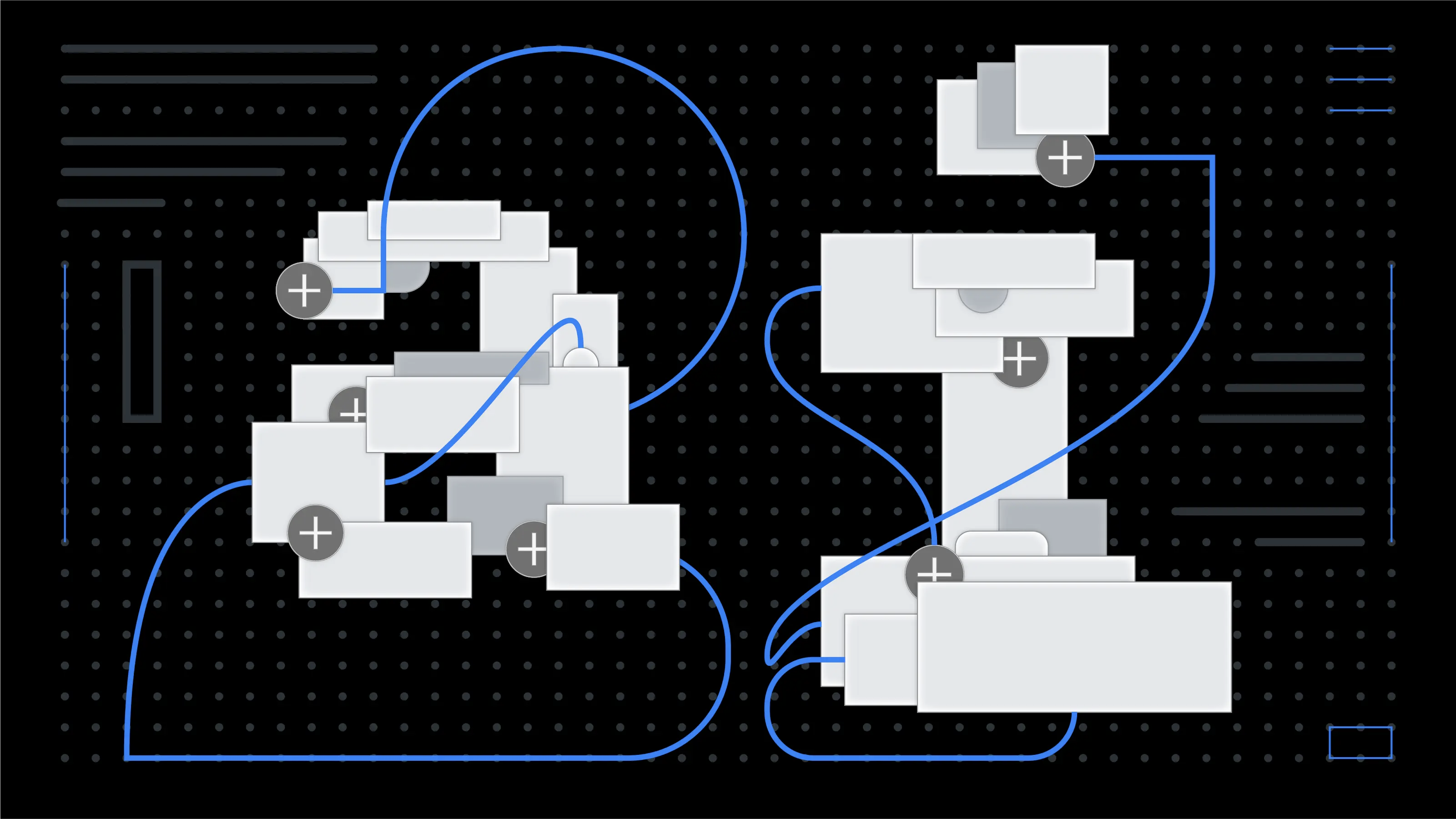
AI Hardware Composer launches on two-year anniversary of IBM AI Hardware Center
14-April-2021
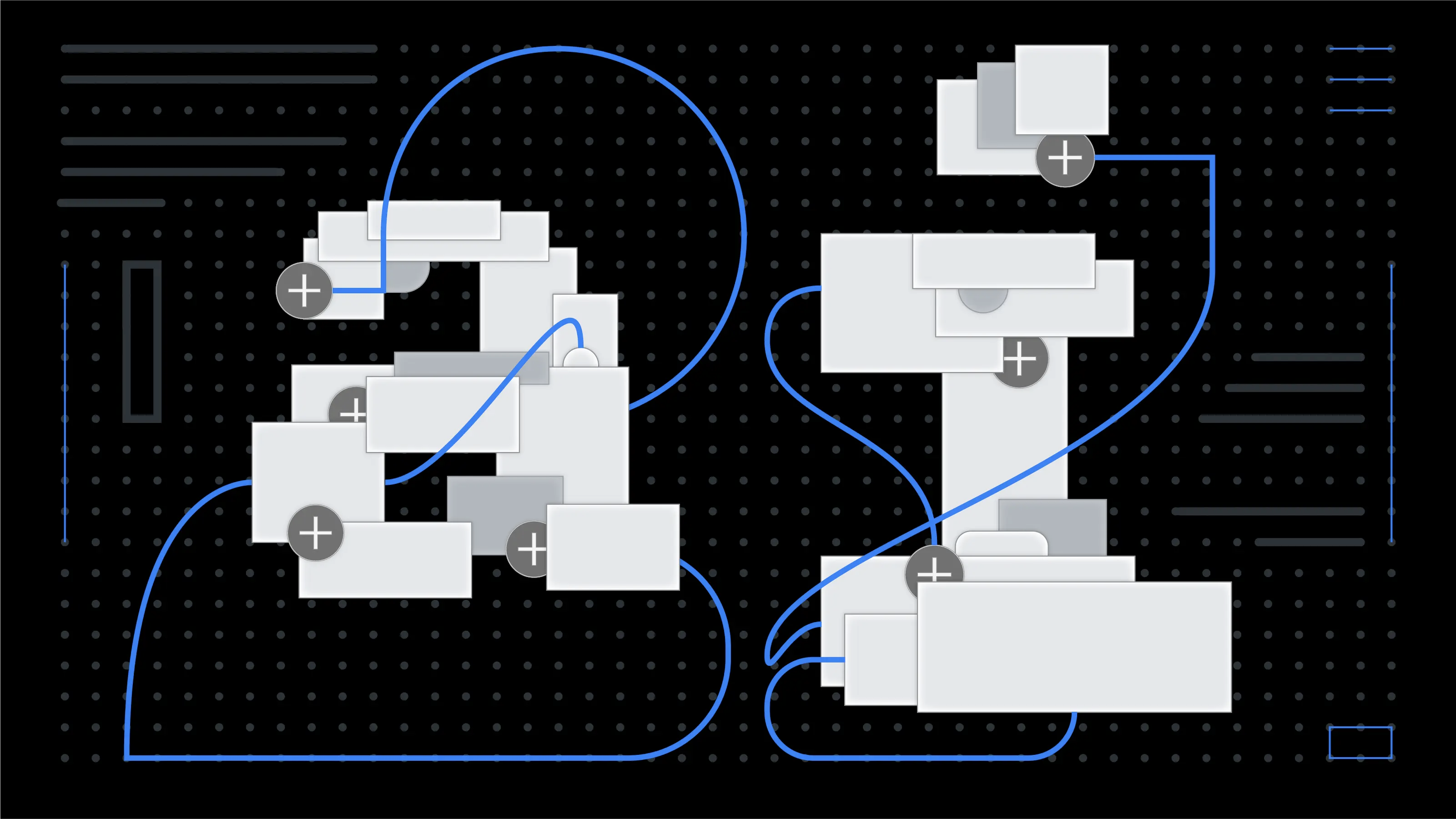
Blog

12 new Project Debater AI technologies available as cloud APIs
17-March-2021

Blog
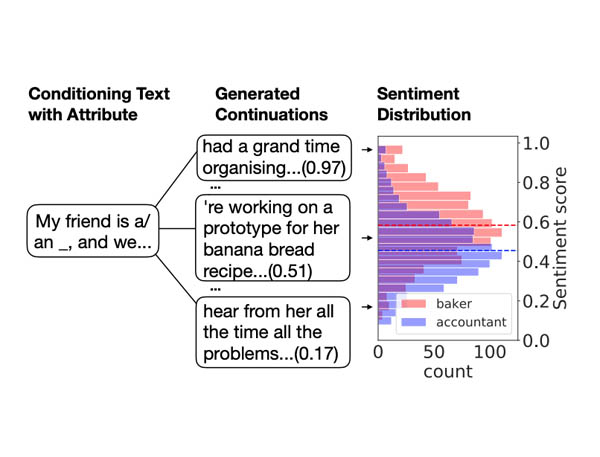
IBM researchers check AI bias with counterfactual text
5-Feb-2021
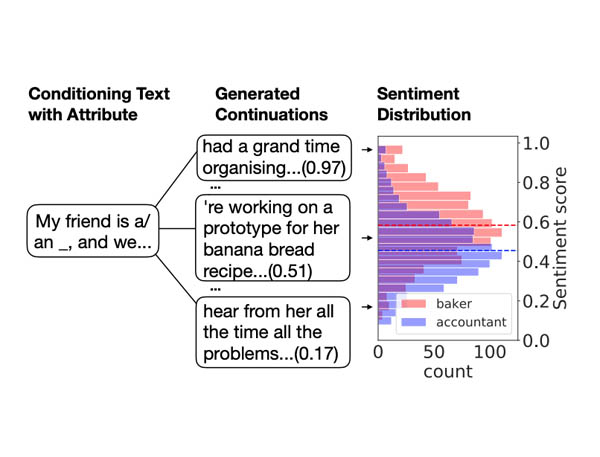
Experiments
A no-code sandbox to experiment with neural network design, and analog devices, and algorithmic optimizers to build high accuracy deep learning models.
The APIs include natural language understanding capabilities that deal with wikification, semantic relatedness between Wikipedia concepts, short text clustering, and common theme extraction for texts.
The Open Source python toolkit for exploring and using the capabilities of in-memory computing devices in the context of artificial intelligence.
Compare VSRL with traditional reinforcement learning to see how they perform under different environmental conditions and with different amounts of training.
Featured publications
Date | Content | Title | Journal / Venue |
---|---|---|---|
March 2021 | Paper |
An autonomous debating system |
Nature |
Jan 2020 | Paper |
Mapping the Space of Chemical Reactions Using Attention-Based Neural Networks |
Nature Machine Intelligence (2021) |
Oct 2020 | Paper |
Text-based RL Agents with Commonsense Knowledge: New Challenges, Environments and Baselines |
AAAI (2021) |
May 2020 | Paper |
Generate Your Counterfactuals: Towards Controlled Counterfactual Generation for Text |
AAAI (2021) |
AI research teams
AI Hardware | |
Algorithmic Acceleration | |
Auto AI (tools) | |
Computer Vision | |
Explainability | |
Fairness | |
Knowledge and Reasoning |
Machine Learning | |
Natural Language | |
Process Automation | |
Robustness | |
Speech | |
Transparency and Accountability | |
Value Alignment |