Computer Vision
Computer Vision
Our research interests include learning with limited labels, cross-domain, self-supervised and multi-modal learning, and modern model architectures. We focus on innovative state-of-the-art research that makes a difference.
Read more about Computer Vision at IBM Research - Israel
Read more about Medical Imaging Analytics and Solutions at IBM Research - Israel
Our research interests include learning with limited labels, cross-domain, self-supervised and multi-modal learning, and modern model architectures. We focus on innovative state-of-the-art research that makes a difference.
Read more about Computer Vision at IBM Research - Israel
Read more about Medical Imaging Analytics and Solutions at IBM Research - Israel
Collaboration & Publications
Publications that were published during 2021
Title | Author | Year | Conference/Journal | Area | |
---|---|---|---|---|---|
Fine-grained Angular Contrastive Learning with Coarse Labels | Guy Bukchin, Eli Schwartz, Kate Saenko, Ori Shahar, Rogerio Feris, Raja Giryes, Leonid Karlinsky | 2021 | CVPR | Self-Supervised Learning, Few-Shot Learning, Few-Shot Classification | Link |
StarNet: towards weakly supervised few-shot detection and explainable few-shot classification | Leonid Karlinsky*, Joseph Shtok*, Amit Alfassy*, Moshe Lichtenstein*, Sivan Harary, Eli Schwartz, Sivan Doveh, Prasanna Sattigeri, Rogerio Feris, Alexander Bronstein, Raja Giryes | 2021 | AAAI | Few-Shot Learning, Few-Shot Classification, Few-Shot Detection | Link |
MetAdapt: Meta-Learned Task-Adaptive Architecture for Few-Shot Classification | Sivan Doveh, Eli Schwartz, Chao Xue, Rogerio Feris, Alex Bronstein, Raja Giryes, Leonid Karlinsky | 2021 | Pattern Recognition Letters | Few-Shot Learning, Few-Shot Classification | Link |
Dynamic Distillation Network for Cross-Domain Few-Shot Recognition with Unlabeled Data | A Islam, CF Chen, R Panda, L Karlinsky, R Feris, RJ Radke | 2021 | NeurIPS | Few-Shot Learning, Few-Shot Classification, Cross-domain Few-Shot Learning | Link |
Detector-Free Weakly Supervised Grounding by Separation | Assaf Arbelle, Sivan Doveh, Amit Alfassy, Joseph Shtok, Guy Lev, Eli Schwartz, Hilde Kuehne, Hila Barak Levi, Prasanna Sattigeri, Rameswar Panda, Chun-Fu Chen, Alex Bronstein, Kate Saenko, Shimon Ullman, Raja Giryes, Rogerio Feris, Leonid Karlinsky | 2021 | ICCV | Text grounding in images | Link |
A Broad Study on the Transferability of Visual Representations with Contrastive Learning | Ashraful Islam, Chun-Fu Chen, Rameswar Panda, Leonid Karlinsky, Richard Radke, Rogerio Feris | 2021 | ICCV | Transfer Learning | Link |
Adafuse: Adaptive temporal fusion network for efficient action recognition | Yue Meng, Rameswar Panda, Chung-Ching Lin, Prasanna Sattigeri, Leonid Karlinsky, Kate Saenko, Aude Oliva, Rogerio Feris | 2021 | ICLR | Action Recognition, Efficient AI | Link |
CHARTER: heatmap-based multi-type chart data extraction | Joseph Shtok, Sivan Harary, Ophir Azulai, Adi Raz Goldfarb, Assaf Arbelle, Leonid Karlinsky | 2021 | KDD DIW | Charts analysis | |
Noise estimation using density estimation for self-supervised multimodal learning | E Amrani, R Ben-Ari, D Rotman, A Bronstein | 2021 | AAAI | Self-Supervised multimodal learning | Link |
More publications
Title | Author | Year | Conference/Journal | Area | |
---|---|---|---|---|---|
Ar-net: Adaptive frame resolution for efficient action recognition Authors | Yue Meng, Chung-Ching Lin, Rameswar Panda, Prasanna Sattigeri, Leonid Karlinsky, Aude Oliva, Kate Saenko, Rogerio Feris | 2020 | ECCV | Action Recognition, Efficient AI | Link |
OnlineAugment: Online data augmentation with less domain knowledge | Zhiqiang Tang, Yunhe Gao, Leonid Karlinsky, Prasanna Sattigeri, Rogerio Feris, Dimitris Metaxas | 2020 | ECCV | Transfer Learning | Link |
TAFSSL: Task-Adaptive Feature Sub-Space Learning for few-shot classification | Moshe Lichtenstein, Prasanna Sattigeri, Rogerio Feris, Raja Giryes, Leonid Karlinsky | 2020 | ECCV | Few-Shot Learning, Few-Shot Classification, Semi-Supervised Learning, Transductive Learning | Link |
A broader study of cross-domain few-shot learning | Yunhui Guo, Noel C Codella, Leonid Karlinsky, James V Codella, John R Smith, Kate Saenko, Tajana Rosing, Rogerio Feris | 2020 | ECCV | Few-Shot Learning, Few-Shot Classification, Cross-domain Few-Shot Learning | Link |
A Maximal Correlation Approach to Imposing Fairness in Machine Learning | Joshua Lee, Yuheng Bu, Prasanna Sattigeri, Rameswar Panda, Gregory Wornell, Leonid Karlinsky, Rogerio Feris | 2020 | arXiv preprint arXiv:2012.15259 | Fairness | Link |
Baby steps towards few-shot learning with multiple semantics | Eli Schwartz*, Leonid Karlinsky*, Rogerio Feris, Raja Giryes, Alex M Bronstein | 2019 | arXiv preprint arXiv:1906.01905 | Few-Shot Learning, Few-Shot Classification, Multimodal Learning | Link |
A CNN based method for automatic mass detection and classification in mammograms | Ayelet Akselrod-Ballin, Leonid Karlinsky, Sharon Alpert, Sharbell Hashoul, Rami Ben-Ari, Ella Barkan | 2019 | Computer Methods in Biomechanics and Biomedical Engineering: Imaging & Visualization (CMBBE) | Medical Vision | |
Repmet: Representative-based metric learning for classification and few-shot object detection | Leonid Karlinsky*, Joseph Shtok*, Sivan Harary*, Eli Schwartz*, Amit Aides, Rogerio Feris, Raja Giryes, Alex M Bronstein | 2019 | CVPR | Few-Shot Learning, Few-Shot Detection | Link |
Laso: Label-set operations networks for multi-label few-shot learning | Amit Alfassy*, Leonid Karlinsky*, Amit Aides, Joseph Shtok, Sivan Harary, Rogerio Feris, Raja Giryes, Alex M Bronstein | 2019 | CVPR | Transfer Learning, Few-Shot Learning, Few-Shot Classification | Link |
Co-regularized alignment for unsupervised domain adaptation | Abhishek Kumar, Prasanna Sattigeri, Kahini Wadhawan, Leonid Karlinsky, Rogerio Feris, Bill Freeman, Gregory Wornell | 2018 | NeurIPS | Domain Adaptation | Link |
Delta-encoder: an effective sample synthesis method for few-shot object recognition | Eli Schwartz*, Leonid Karlinsky*, Joseph Shtok, Sivan Harary, Mattias Marder, Abhishek Kumar, Rogerio Feris, Raja Giryes, Alex Bronstein | 2018 | NeurIPS | Few-Shot Learning, Few-Shot Classification | Link |
Domain specific convolutional neural nets for detection of architectural distortion in mammograms | Rami Ben-Ari, Ayelet Akselrod-Ballin, Leonid Karlinsky, Sharbell Hashoul | 2017 | ISBI | Medical Vision | Link |
Fine-grained recognition of thousands of object categories with single-example training | Leonid Karlinsky, Joseph Shtok, Yochay Tzur, Asaf Tzadok | 2017 | CVPR | Few-Shot Learning, Few-Shot Classification, Few-Shot Detection | Link |
Hybrid remote expert-an emerging pattern of industrial remote support | Ethan Hadar, Joseph Shtok, Benjamin Cohen, Yochay Tzur, Leonid Karlinsky | 2017 | CAISE 2017 | AR | Link |
Deep learning for automatic detection of abnormal findings in breast mammography | Ayelet Akselrod-Ballin, Leonid Karlinsky, Alon Hazan, Ran Bakalo, Ami Ben Horesh, Yoel Shoshan, Ella Barkan | 2017 | Deep learning in medical image analysis and multimodal learning for clinical decision support | Medical Vision | |
A region based convolutional network for tumor detection and classification in breast mammography | Ayelet Akselrod-Ballin, Leonid Karlinsky, Sharon Alpert, Sharbell Hasoul, Rami Ben-Ari, Ella Barkan | 2016 | Deep learning and data labeling for medical applications (DLDL) | Medical Vision | |
Deep Learning and Data Labeling for Medical Applications | A Akselrod-Ballin, L Karlinsky, S Alpert, S Hasoul, R Ben-Ari, E Barkan, G Carneiro | 2016 | Int. Work. Large-Scale Annot. Biomed. Data Expert Label Synth | Medical Vision |
Workshops & Tutorials
Women in AI Israel (2019, 2020, 2021)
A community-driven initiative aimed at increasing the number of women working in AI and introducing the AI community to female researchers & practitioners.
VL3: Visual Learning with Limited Labels.
VISUAL LEARNING WITH LIMITED LABELED DATA
Open Source Activities
LASO This repository contains the implementation of "LaSO: Label-Set Operations networks for multi-label few-shot learning" by Alfassy et al. It was posted on arxiv in Feb 2019 and will be presented in CVPR 2019. |
|
RepMet RepMet few-shot detection engine and an Imagenet-LOC detection benchmark |
|
DeltaEncoder Implementation for the paper "Delta-encoder: an effective sample synthesis method for few-shot object recognition" from NeurIPS 2018 |
|
Cross-Domain Few-Shot Learning (CD-FSL) Benchmark Cross-Domain Few-Shot Learning (CD-FSL) Benchmark |
|
Multi-scale, Multi-object Star Model detection algorithm Multi-scale multi-object star model Matlab code for single shot object detection & recognition. Trained using one or few examples per object, can support up to few thousands of objects at once. |
ANCOR Fine-grained Angular Contrastive Learning with Coarse Labels (CVPR 2021 Oral) |
|
TAFSSL Task-Adaptive Feature Sub-Space Learning for few-shot classification |
|
AR-Net Adaptive Resolution Network for Efficient Video Understanding |
|
Online-augment Official OnlineAugment implementation in PyTorch |
|
AdaFuse Adaptive Temporal Fusion Network for Efficient Action Recognition (ICLR 2021) |
Awards
Eli Schwartz (Tel Aviv University):
IBM PhD Fellowship (2020)
Eli Schwartz has received the IBM PhD Fellowship, a competitive award given to PhD students who have demonstrated academic excellence as well as provided innovative, exceptional research proposals.
IBM Research Outstanding Innovation Award (OIA) 2020
Contribution to science in the Learning with Less Labels (LwLL) domain – data limited learning for vision and beyond” science accomplishment for the year 2020. This accomplishment is a cross-lab effort. The total contributions included: 16 papers with over 400 citations, 17 patents, and 3 successful customer engagements, as well as numerous academy collaborations.
IBM Research Dynamic Neural Networks for Efficient AI (OIA) 2020
(Non-invited) Venturebeat article published by an independent journalist and describing Leonid’s StarNet AAAI 2021 paper. Through this article IBM’s explainable AI toolkit gets promoted and compared as equal to similar efforts by Facebook, Microsoft, and Google.
Task Adaptive Feature Sub-Space Learning (TAFSSL)
In this work, we explored ways of how unlabeled data of different kinds can be leveraged to significantly boost few-shot learning performance.
Our paper TAFSSL (listed as ICA + MSP) is #1 for 1-shot (were #1 in 2020)
We are #3 in 1-shot (were #1 in 2020)
Talks
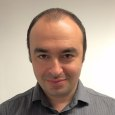
Leonid Karlinsky
Explainable, Adaptive, and Cross-Domain Few-Shot Learning
Talk for the 2d3d.ai meetup 2021
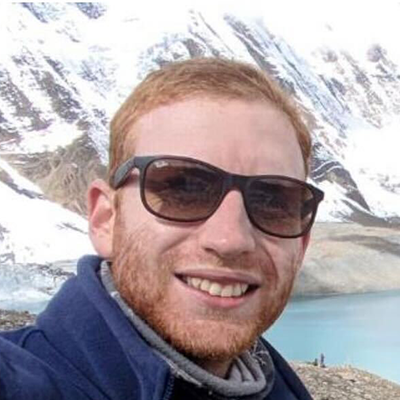
Guy Bbukchin (Intern)
Fine-grained Angular Contrastive Learning with Coarse Labels
Oral Session for CVPRi 2020 (hebrew)
More talks
Sivan Doveh - Meta-learning: few-shot learning and neural architecture search,Talk for the Deep Corona Academy - Alibaba meetup
Eli Schwartz - Small-Data in the Big-Data Era, Talk, The 6th Ambassadors' Summit, Tel Aviv University
Leonid Karlinsky - ECCVi 2020 (hebrew)
Amit Alfassy - LaSO: Label-Set Operations networks for multi-label few-shot learning, Oral Session for CVPR 2019.
Leonid Karlinsky - IMVC 2019
Eli Schwartz - Few-shot learning with multiple semantics, CVPR 2019 Language And Vision Workshop
Leonid Karlinsky - Data Science Summit 2018
Eli Schwartz - Presenting our NeurIPS 2018 paper
Eli Schwartz - Few-shot learning, at the Geometric Computing Lab, Stanford University, USA, October 2018
Let's talk
Want to collaborate? We're always happy to talk. Feel free to get in touch.
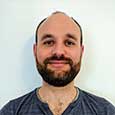
Assaf Arbelle
Computer vision Area Focal Point
Working together
with IBM Research - Israel
Our published papers
Our catalog of recent publications authored by IBM researchers, in collaboration with the global research community.
Open positions and internships
Join some of the best scientists from around the world, at IBM Research - Israel. This is a list of the current open positions, including internships and and student opportunities.
Meet our interns
Explore
Grants &
fellowship
IBM supports students in their research, encourages and invites the Fellowship winners to do an internship in order to be better informed with the the research done by the company.
EU funded
projects
IBM Research - Israel is involved in over 30 EU-funded consortiums and conducts numerous joint projects with academic and industrial partners around the world.