IBM Research - Israel
Accelerated Discovery
We are developing causal inference technologies and multimodal learning models that leverage the most advanced deep learning and generative models, aimed at accelerating molecules and biomarker discovery.
News and Blogs
BICauseTree: Bias-balancing Interpretable Causal Tree
In this blog post, ISRL intern Lucile Ter-Minassian outlines a binary decision tree model for causal inference that interprets Average Treatment Effects (ATEs) without bias from high-dimensional datasets.
Impact of COVID-19 on Clinical Findings in Medical Imaging Exams
This study, coauthored by ISRL researchers, examines the increase in the rate of abnormal findings in MR-MSK and CT-brain exams after the onset of the COVID-19 pandemic.
IBM and Cleveland Clinic unveil the first quantum computer dedicated to healthcare research
Research projects by ISRL researchers Yishai Shimoni, Michael Danziger, and Liran Szlak were profiled in this blog post about IBM's partnership with Cleveland Clinic.
Causal Inference is a Mindset
Researcher Ehud Karavani blogs about the philosophical challenges and a myriad of practical tools as we strive for causal inference from observational data.
Research Projects
Causal Machine Learning for Healthcare & Life Sciences |
The Causal Machine-Learning for Healthcare and Life-Sciences team includes researchers from the fields of physics, computer science, statistics, and epidemiology. We conduct analyses for many types of healthcare-related data, including electronic health records and insurance claims data holding the medical history of over 150 million people. |
Multimodal AI for Healthcare & Life Sciences |
Our research includes advanced computer vision techniques that enable the automatic extraction of diagnostically relevant features in multimodal healthcare images. We are developing machine learning tools that combine multimodal semantic image descriptions (for mammography, ultrasound and MRI) with clinical data to help estimate a correct differential diagnosis and support patient management recommendations. |
Publications
IBM researchers in Israel publish a wide variety of work every year as part of their work on research projects in the lab, in collaboration with other researchers and scientists in IBM, and together with academic and industrial partners from around the world. Researchers in our group publish works at conferences and in scientific journals such as the SPIE Medical Imaging conferences, Frontiers in Pharmacology, Radiology, and others. |
Tools & Code
Causal Inference 360 Open Source Toolkit
This open source Python toolkit is designed to bring long-standing machine-learning methodologies to the field of causal inference.
fuse-med-ml
FuseMedML is an open-source Python-based framework designed to enhance collaboration and accelerate discoveries in fused medical data through advanced machine learning technologies. This initial version is PyTorch-based and focuses on deep learning for medical imaging and digital pathology.
Academic Collaboration
Collaborate with our researchers in accelerating scientific discoveries in the medical domain - from drug discovery to biomarker discovery - using AI-based technology applied to health, chemical, and molecular data, embedded with medical knowledge. |
Let's talk
We're always happy to talk. Feel free to get in touch.
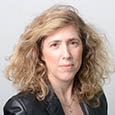
Michal Rosen-Zvi
Manager, Accelerated Discovery, IBM Research - Israel